Notre équipe
L'équipe METHODS est une équipe pluridisciplinaire composée d'épidémiologistes, de statisticiens, de mathématiciens, de data-scientists et de cliniciens dans divers domaines (psychiatrie, nutrition, maladies respiratoires, cancer digestif, etc.)
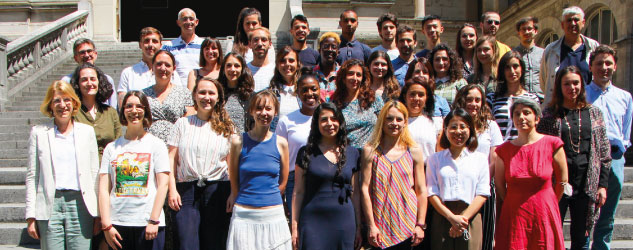
Notre recherche
Nos objectifs sont de repenser l'évaluation thérapeutique des maladies chroniques, de proposer de nouveaux concepts et de développer de nouvelles approches méthodologiques.
Notre recherche s'articule autour de 4 axes :
- Production de données probantes (responsable : Viet-Thi Tran)
- Médecine personnalisée (responsable : Raphaël Porcher)
- Synthèse des données probantes (responsable : Anna Chaimani)
- Recherche sur la recherche (responsables : Isabelle Boutron, Philippe Ravaud).
L'équipe est adossée au Centre français EQUATOR (Directeur P. Ravaud) et Cochrane France (Directrice I. Boutron), ce qui lui confère une position stratégique au niveau international.
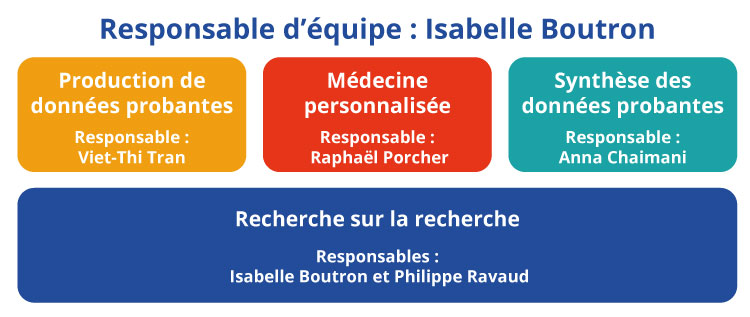
Notre expertise
Notre équipe est reconnue pour son expertise en :
- Sciences participatives pour passer à un modèle où les décisions de recherche sont prises par tous les acteurs de la maladie (patients, médecins, etc.). Nous avons été pionniers dans l’utilisation de ces méthodes pour développer des 'Core Outcome Sets'.
- Santé numérique. Nous travaillons sur les méthodes d'évaluation des interventions numériques, en particulier interventions thérapeutiques digitales et 'Just in time adaptative interventions' (JITAI). Nous explorons les modalités optimales d'intégration de la médecine digitale dans un soin centré sur le patient. Nous dirigeons la partie académique de @Hôtel-Dieu, un ambitieux partenariat public-privé visant à développer une interface entre les organisations publiques et les start-ups technologiques.
- Médecine personnalisée. Nous développons des méthodes innovantes pour identifier les patients les plus susceptibles de répondre à un traitement en tenant compte de l'efficacité et de la tolérance et proposons de nouveaux plans expérimentaux d’ essais cliniques pour la médecine personnalisée.
- Plans expérimentaux innovants. Nous avons développé la plateforme CORIMUNO dans laquelle 12 essais cliniques randomisés ont été menés (1238 patients, 34 hôpitaux). Nous avons aussi mené une série d'essais émulés en utilisant des données collectées en routine pour évaluer l'efficacité de médicaments prescrits à titre compassionnel (ex: hydroxychloroquine évaluée avant les résultats des premiers essais randomisés).
- Synthèse des données factuelles, méta-analyses en réseau, méta-analyses 'vivantes'. Nous développons des méthodes pour prendre en compte différentes types d’études (etudes randomisés / études observationnelles), et l'évolution temporelle des traitements. Nous avons mis en œuvre l’initiative internationale COVID-NMA qui a mis à disposition des décideurs une cartographie des essais et une revue systématique 'vivantes' des données probantes, mise à jour en temps réel (>800 essais analysés). La plateforme a été soutenue par l'OMS et identifiée comme une source d'information importante pour les cliniciens et les décideurs par la Commission du Lancet dédiée aux leçons à tirer de la pandémie de COVID-19.
- Recherche sur la Recherche interventionnelle pour explorer les moyens de transformer la planification, la mise en oeuvre et la communication des résultats de la recherche, ainsi que le processus d'évaluation par les pairs. Nous avons été pionnier dans l’exploration du concept de "spin" (i.e., distorsion de la présentation et interprétation des résultats de la recherche) et nous sommes internationalement reconnus pour notre travail en science ouverte via notamment l'élaboration et l’implémentation de recommandations visant à améliorer la transparence et la reproductibilité de la recherche (ex : CONSORT Statement).
Collaborations internationales
Nous collaborons étroitement avec plusieurs équipes reconnues dans notre domaine et nous faisons partie du comité de pilotage de réseaux internationaux importants, notamment :
- Le réseau EQUATOR - Enhancing the QUAlity and Transparency Of health Research
- Le comité de pilotage SPIRIT-CONSORT
- Le groupe Cochrane Bias Methods
Coordonnées et localisation
Hôtel-Dieu
1 place du Parvis Notre-Dame
75004 Paris
sec.epidemio.htd@aphp.fr